Summary
Disclaimer: This summary has been generated by AI. It is experimental, and feedback is welcomed. Please reach out to info@qconlondon.com with any comments or concerns.
The presentation titled "Foundation Models for Ranking: Challenges, Successes, and Lessons Learned" by Moumita Bhattacharya discusses the development and application of foundation models in search and recommendation systems, particularly at Netflix.
Main Topics Covered:
- The Role of Recommender Systems: Emphasized as key drivers for user engagement in modern products such as streaming services and e-commerce platforms.
- Two-Stage Ranking Approach: Described as the method of handling large catalogs by initially narrowing down search results before applying complex ranking models.
Foundation Models:
- Development of a unified contextual model called Unicorn to power Netflix's search and recommendation systems.
- Use of large transformer-based models to capture user preferences and improve personalization efficiency.
Challenges & Solutions:
- Training Optimization: Addressing the need for efficient algorithms due to the large size of foundation models.
- Computational Resource Management: Optimizing GPU usage and data processing to support large-scale training.
- Balancing Personalization with Relevance: Ensuring search results align with both user preferences and semantic content.
Outcomes and Benefits:
- Unified Model Advantages: Reducing maintenance costs and improving innovation across different product areas.
- Enhanced Recommendation Results: Achieved significant improvements in off-line and online testing metrics.
Conclusion:
The presentation highlighted the potential of foundation models to transform recommendation systems by integrating large-scale data-driven algorithms. The challenges faced, such as resource allocation and maintaining balance between personalization and relevance, provide valuable lessons for implementing large models effectively in industry settings.
Speaker's Background: Moumita Bhattacharya leads the Foundation Models Development team at Netflix, bringing her experience from her previous roles at Etsy and a strong academic background in machine learning.
This is the end of the AI-generated content.
Abstract
Recommender systems are an integral part of most products nowadays and are often a key driver of discovery for users of the product. Developing a large-scale recommender system that can provide personalized recommendations to several hundred million users while ensuring relevance and timeliness is as much of a machine-learning problem as it is an engineering task. Additionally, with the recent advancement in the AI space, we are able to train larger and larger models that can capture users' long-term preferences, which ultimately improves the personalization of such recommender systems. However, building and integrating such large foundation models into the recommender systems is yet another machine learning and engineering task that requires a lot of optimization of the algorithms, as well as the training and inference process.
In this talk, I will cover building such Recommender Systems and Foundation Models that can power products for multi-million users as well as highlight challenges faced, things to be considered, and success stories in real-world applications. This talk will bring the engineering and infrastructure perspective on these tasks, while also highlighting the AI and machine learning perspective.
Speaker
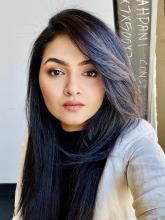
Moumita Bhattacharya
Senior Research Scientist @Netflix, Previously @Etsy
Moumita Bhattacharya leads the Foundation Models Development team at Netflix, where she manages a team of research scientists and software engineers. Her work focuses on developing large-scale foundation models for Search and Recommendation Systems. Prior to Netflix, she was a Senior Applied Scientist at Etsy, where she was tech leading a team that developed recommendation systems to show relevant products to Etsy users. Moumita has a PhD in Computer Science with a focus on Machine Learning and its applications. She has been actively serving as Program Committees for WebConf and is a reviewer for conferences such as RecSys, SIGIR, WebConf, AAAI, and various journals. Moumita is also an adjunct faculty in the Data Science Institute (DSI) of the University of Delaware.