In a world powered by data, crafting the right architecture has never been more critical—or more complex. With an ever-growing arsenal of tools and solutions, designing a robust, future-ready data architecture requires not just expertise, but pragmatism and foresight. The challenge? Building scalable systems that meet the demands of today while anticipating the needs of tomorrow—especially when AI and machine learning enter the mix.
As companies embrace Data Mesh architectures to decentralize data ownership, they face a cascade of hurdles: scaling infrastructure, integrating real-time data streams, ensuring data governance, and automating agile data pipelines—all while keeping costs in check. The race to innovate is on, and the winners will be those who can turn these challenges into opportunities.
Join us at our Modern Data Architectures track, where industry experts will share the actionable insights, cutting-edge strategies, and real-world case studies you need to navigate this evolving landscape. Whether you're leading your company's data transformation or fine-tuning your existing architecture, this is your chance to discover the best practices in Modern Data Architecture and becoming best equipped to design a future proof data application.
From this track
Reliable Data Flows and Scalable Platforms: Tackling Key Data Challenges
Wednesday Apr 9 / 10:35AM BST
There are a few common and mostly well-known challenges when architecting for data. For example, many data teams struggle to move data in a stable and reliable way from operational systems to analytics systems.
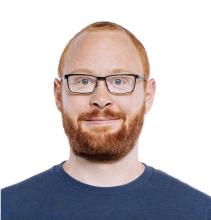
Matthias Niehoff
Head of Data and Data Architecture @codecentric AG, iSAQB Certified Professional for Software Architecture
Achieving Precision in AI: Retrieving the Right Data Using AI Agents
Wednesday Apr 9 / 11:45AM BST
In the race to harness the power of generative AI, organizations are discovering a hidden challenge: precision.
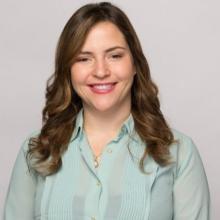
Adi Polak
Director, Advocacy and Developer Experience Engineering @Confluent, Author of "Scaling Machine Learning with Spark" and "High Performance Spark 2nd Edition"
Panel: Modern Data Architectures
Wednesday Apr 9 / 01:35PM BST
The Data Backbone of LLM Systems
Wednesday Apr 9 / 02:45PM BST
Any LLM application has four dimensions you must carefully engineer: the code, data, models and prompts. Each dimension influences the other. That's why you must learn how to track and manage each. The trick is that every dimension has particularities requiring unique strategies and tooling.
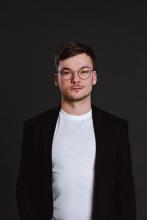
Paul Iusztin
Senior ML/AI Engineer, MLOps, Founder @Decoding ML
Beyond the Warehouse: Why BigQuery Alone Won’t Solve Your Data Problems
Wednesday Apr 9 / 03:55PM BST
Many organizations mistake the adoption of a data warehouse, like BigQuery, as the golden ticket to solving all their data challenges. But without a robust data strategy and architecture, you’re simply shifting chaos into the cloud.
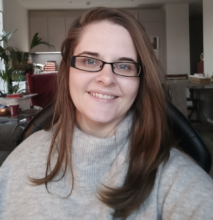
Sarah Usher
Data & Backend Engineer, Community Director, Mentor